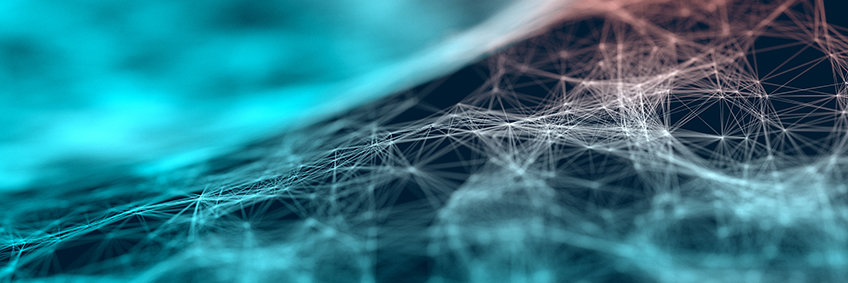
Research Group Statistical Genetics
Bertram Müller-Myhsok
Our group focuses on the quantitative analysis of genetic data and transcriptomic data in psychiatric disease (mostly major depression, but also PTSD and schizophrenia) and its treatment. We also work on metabolomic as well as proteomic data in the same spirit. We are trying to marry statistical genetics and machine learning methods, extending the scope of analysis beyond pure genetics.
Bild: iStock_piranka