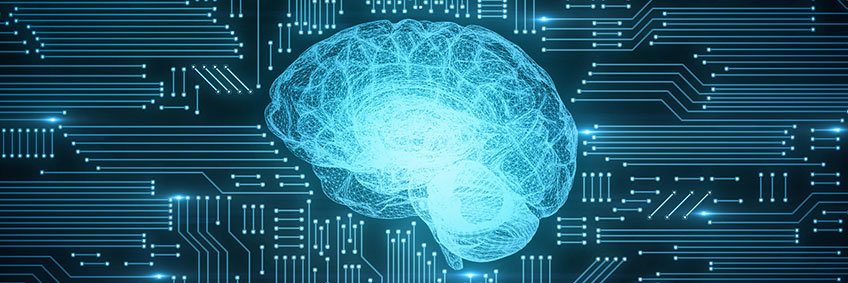
Max Planck Fellow Group Precision Psychiatry
Nikolaos Koutsouleris
The recent years have witnessed the rapid development of computer-assisted diagnostic and prognostic procedures in medicine. Machine learning and AI algorithms are increasingly being used to extract patterns from large and complex databases which, unlike in the past, no longer only describe differences between patient groups or associations between different clinically relevant characteristics but can be used for the prediction of individual disease courses and outcomes. This opens completely new possibilities for personalization in medicine, which allow the individual risk and resource profile of the single patient to be better accommodated in therapy planning than ever before.
In psychiatry in particular, these developments have led to new biomarker-based research approaches that aim to correctly assess the individual risk of developing psychiatric illnesses in the early disease stages and to initiate preventive interventions on the basis of these more precise and earlier predictions. In addition to improving the early detection of mental illnesses, predictive psychiatry endeavours to develop clinical and biological models for a better prediction of individual and differential therapeutic response. Pattern recognition is used to obtain signatures from clinical, neuropsychological, imaging-based and, where appropriate, genetic data that can be applied to individual patients for a quantitative prediction of desired and undesired drug effects. Should these experimental methods prove to be robust and replicable in the next few years, it would be possible to assemble a combination of therapeutic methods that is maximally effective and least burdensome for the individual patient.
Bild: iStock_wigglestick